Appearance
1. 论文
- V1: Going Deeper with Convolutions
- V2: Batch Normalization: Accelerating Deep Network Training by Reducing Internal Covariate Shift
- V3: Rethinking the Inception Architecture for Computer Vision
- V4: Inception-v4, Inception-ResNet and the Impact of Residual Connections on Learning
2. V1
Inception V1主要就是将不同的CONV和POOL堆叠在一起,一方面增加了网络的宽度,一方面提高了尺寸的自适应性,即不需要人为确定应该使用什么尺寸的filter,而是让网络来自己学习。
如上图(a)所示,左边就是一个简单的Inception module,对输入层分别使用
对于其中
此时计算量为
3. V2
V2相比V1主要有以下改进:
- 使用了 Batch Normalization
- 使用两个
的卷积核来代替一个 的,一方面减少了参数,另一方面增加了更多的非线性变换(网络深度)
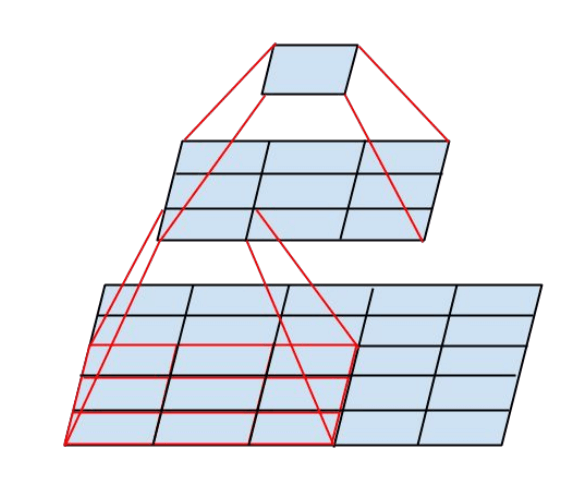
此时 Inception module 如下图所示:
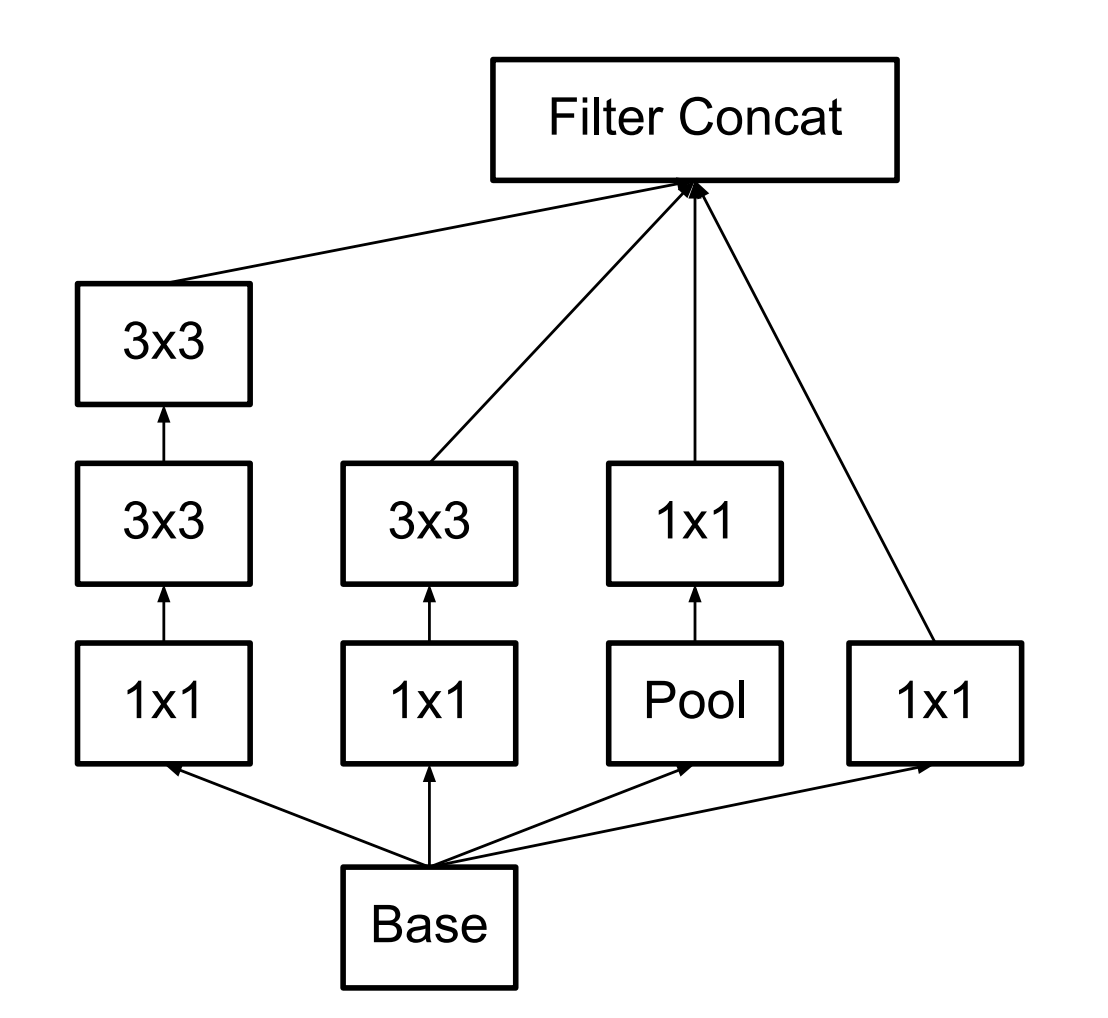
4. V3
V3使用
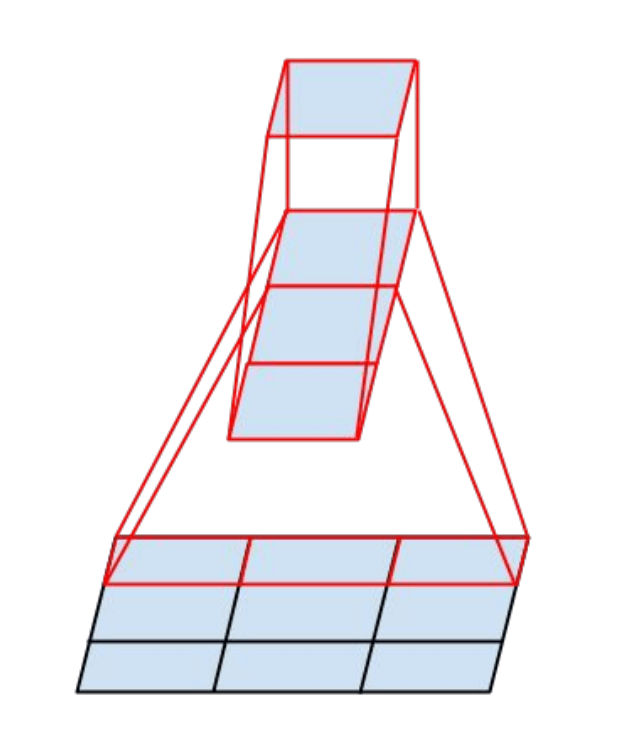
在Inception V3中,有三种Inception module,如下图示:
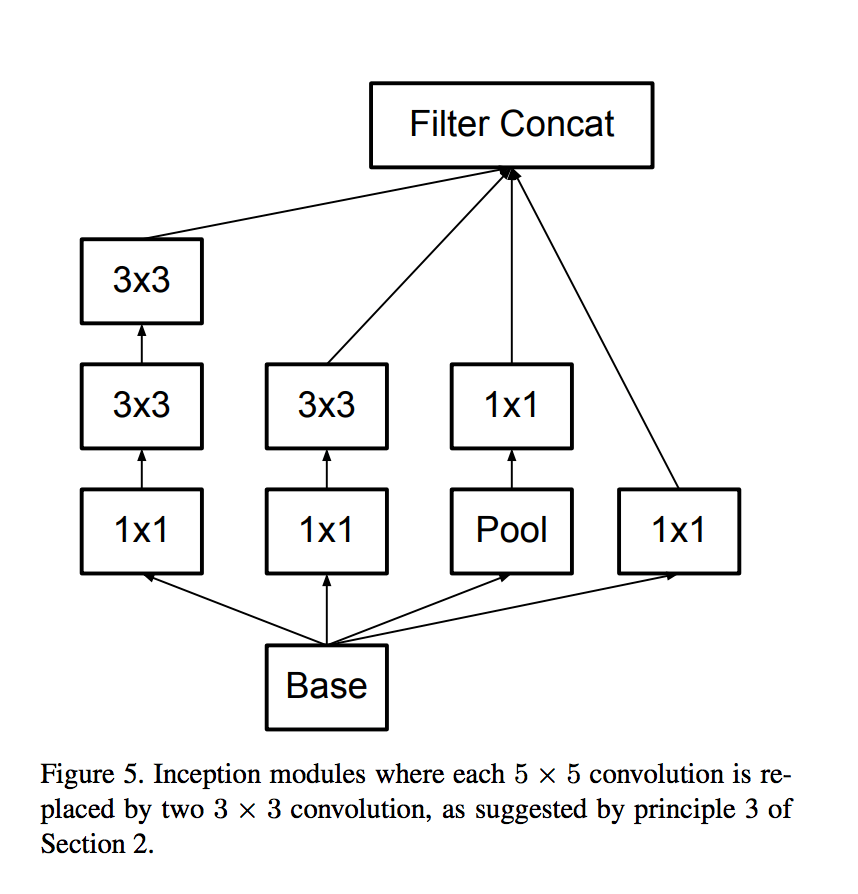
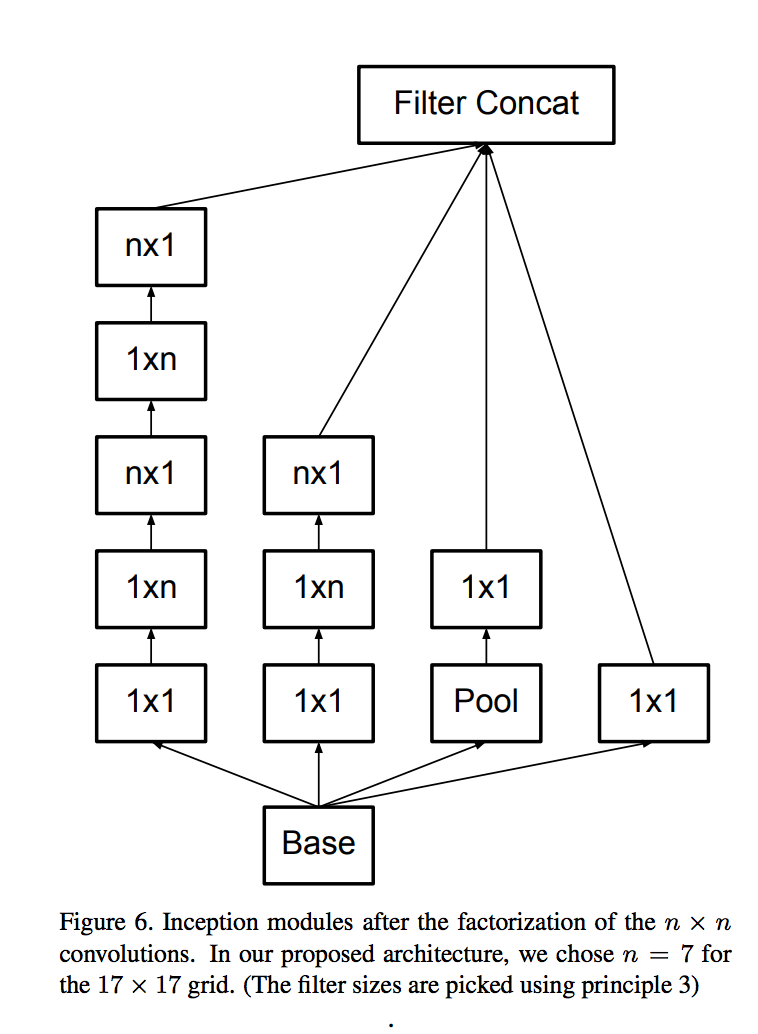
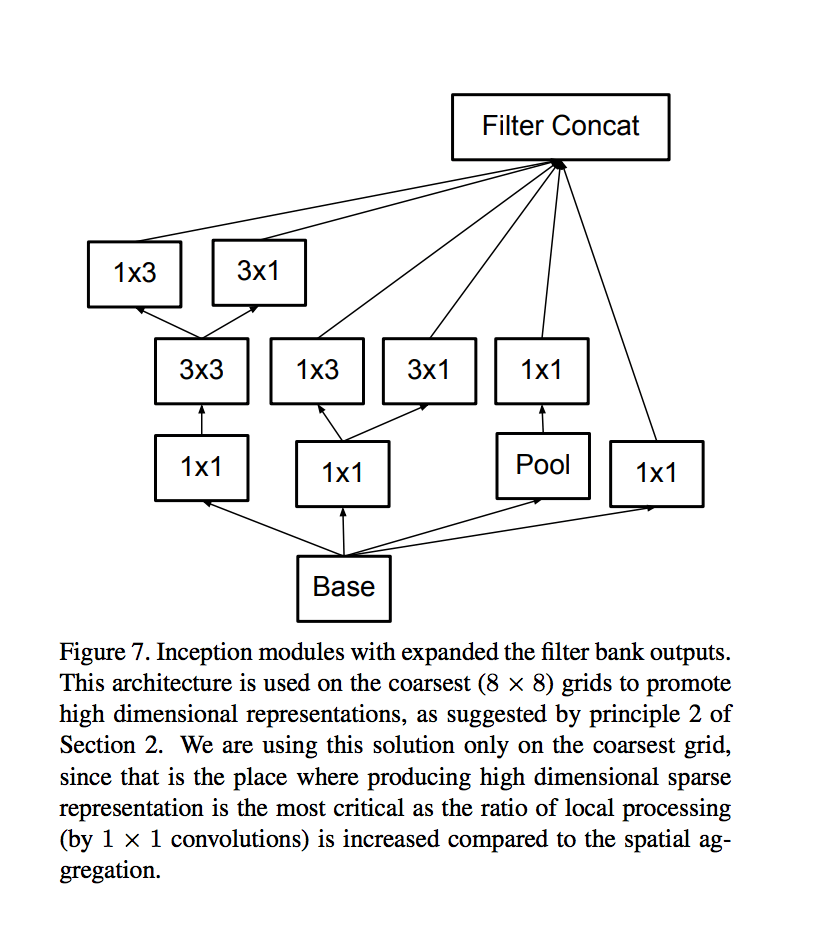
最终的网络结构如下表所示:
5. V4
Inception V4是Inception与ResNet相结合。
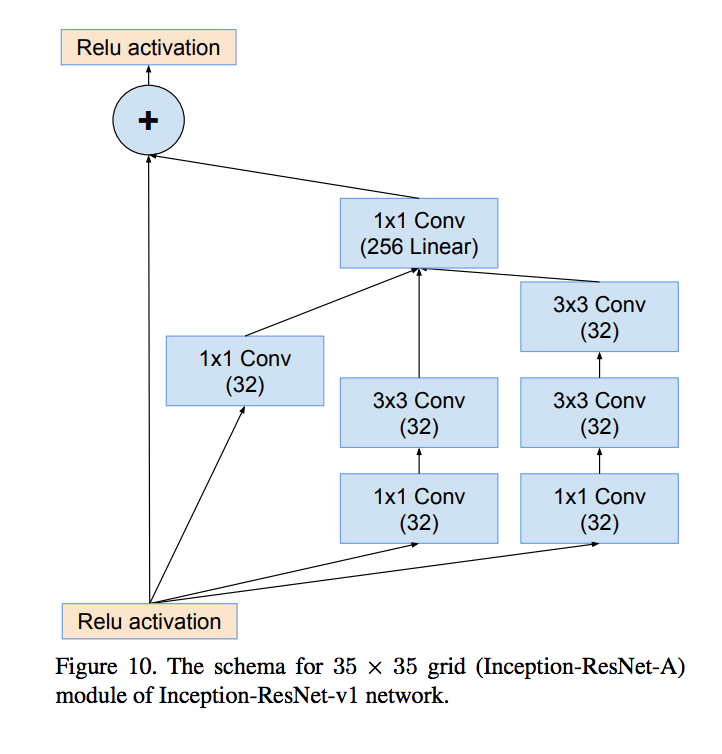
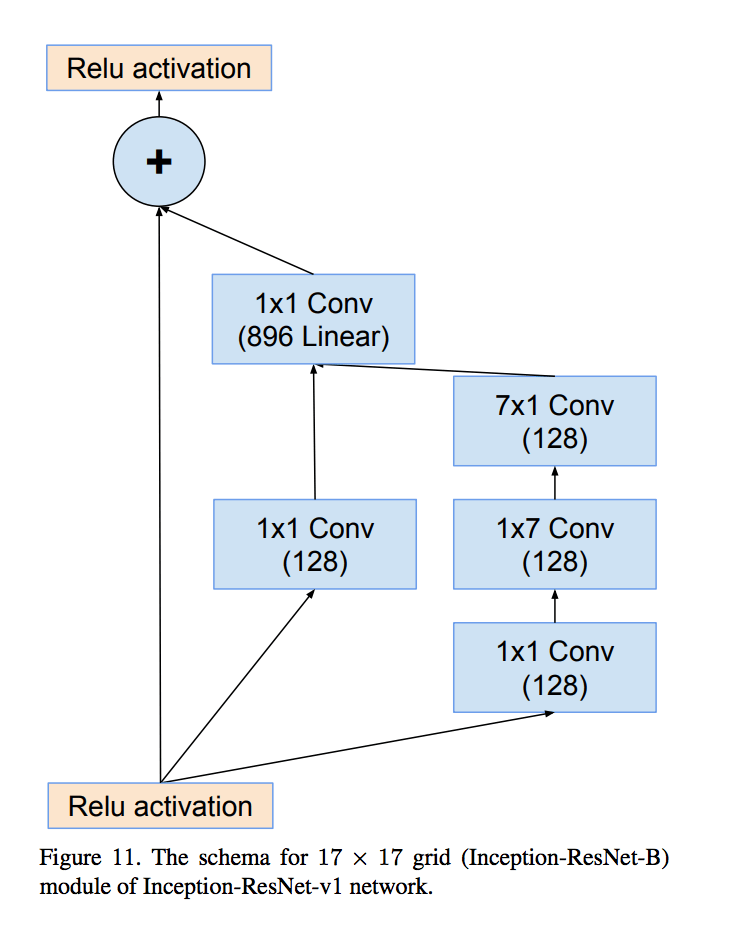
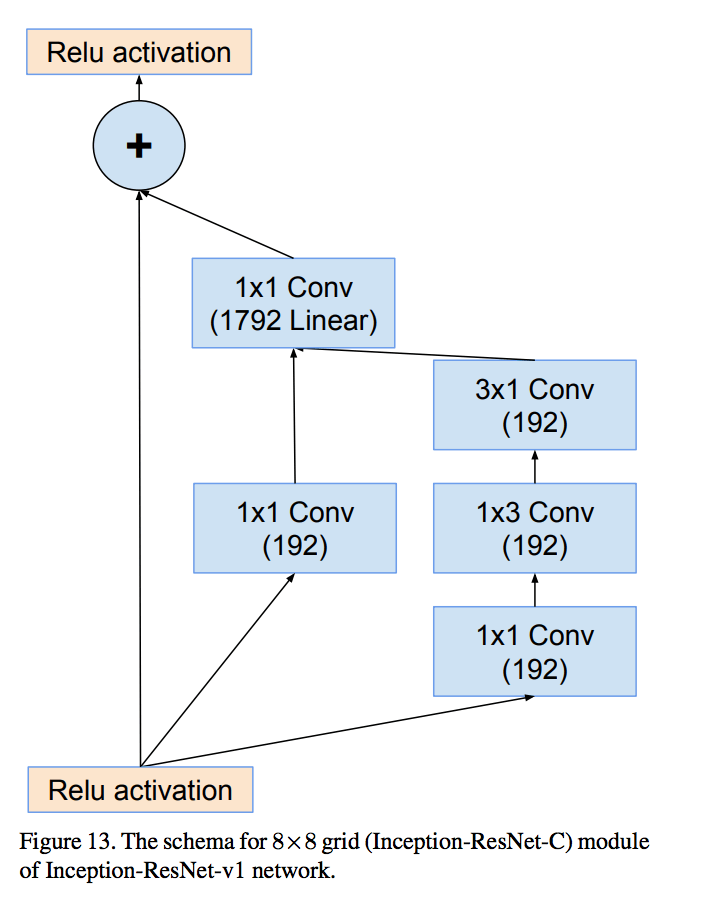